Meet Lassi Paavolainen: From computer science to machine learning for bioimage profiling in health research
The “Behind the Science'' profile series takes an in-depth look at a scientist or group within the Nordic EMBL Partnership. In this article, we meet FIMM’s Academy Research Fellow, Dr. Lassi Paavolainen, and learn about the advances in bioimaging he aims to make as a new group leader at FIMM.
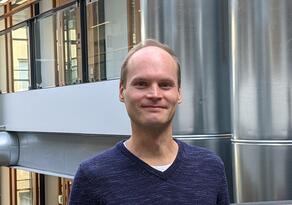
Dr. Lassi Paavolainen was recently awarded an Academy of Finland Research Fellowship supporting the launch of his research group at the Institute for Molecular Medicine Finland (FIMM) in September 2021. Throughout his career Dr. Paavolainen has been fascinated by the wealth of information in bioimages and the power of machine learning to extract that information. Now, with his independent research group, he is able to continue delving into questions about imaging in biology, from an analytical, deep-learning perspective.
Dr. Paavolainen explains, in a nutshell:
My research focuses on both image analysis and machine learning methods for profiling microscopy data. We try to uncover complex information using machine learning. We develop novel deep learning methods, study various approaches to create models, and apply these models to health research-related applications.
While the generalizable methods that he and his group develop might be applicable to any disease model, cancer is his primary focus.
The power of heterogeneity in cancer
There are many reasons why researchers dedicate their careers to studying cancer, and many challenges to fully understanding the disease and contributing to improved diagnosis and treatment. Dr. Paavolainen has a perspective that one may not hear very often – a positive spin on the high amount of heterogeneity of cancer: “cancer is, from an imaging point of view, a very interesting topic, because there is a high heterogeneity of the cells and their interactions, which can be relevant for treatment. To get information for a large landscape of cancer, microscopy methods are essential.”
Work in his group is aimed to improve pathological approaches with tissue sections and to better understand molecular cell biology. Dr. Paavolainen explains:
We are working on a higher level with pathology of tissue sections to go beyond what we can see in cell cultures. We ask questions like ‘what kind of cells are next to each other; what are the interactions; and what kinds of regions are there in the tissue?’ We are also exploring drug screening data and what happens in single cells in culture.
Two complementary arms: method development and application
Dr. Paavolainen’s research program has two main, related arms – machine-learning method development and application in biology and medicine. He explains, “image analysis and machine learning methods are now very much interconnected; there's a rich computer science approach in bioimage profiling.”
On the machine-learning method development side, Dr. Paavolainen’s group builds universal, generalizable and robust machine learning models that can be used with most types of images. He relates it to medical training, “a pathologist learns to identify different types of cells by supervision, but most learning comes with experience (unsupervised approaches); we are not given annotation for everything that we see.”
And, this is a challenge for machine learning where methods traditionally use annotated data.
“It would be very interesting to also discover better ways to create self-supervised approaches, where basically machine learning models learn directly from the data without any expert annotations, or a very small set of pre-given annotations,” further explains Dr. Paavolainen.
On the application side of Dr. Paavolainen’s research program, there are several ongoing projects. For example, a focused machine learning and image analysis approach can be used to examine a region of individual cells, and their nearest neighbors. He illustrates:
Working with Teijo Pellinen at FIMM and Arne Östman at Karolinska Institutet, we just submitted a manuscript of our studies of cancer associated fibroblasts in non-small cell lung cancer. We are studying these fibroblasts, which are in close proximity to cancer cells, and how they can be used as a prognostic biomarker for patient survival.
The heterogeneity of cancer comes into play: “it might be that a larger context is more important for a prognostic biomarker study. We plan to look for wider associations from these microscopy images, not necessarily cells that are simply next to each other,” Dr. Paavolainen explains.
Interdisciplinary collaboration
Considering Dr. Paavolainen’s work with biomedical researchers, it comes as no surprise that he not only appreciates interdisciplinary collaboration but has spent his career fostering a network of such interactions.
Working as a postdoctoral researcher at FIMM, Dr. Paavolainen initially developed key collaborations with Peter Horvath, FIMM group leader and former Tekes FiDiPro fellow, and with Vilja Pietiäinen and Teijo Pellinen, senior researchers in Professor Olli Kallioniemi’s research group. In the years since, he has worked with a team to build up and launch the High Content Imaging and Analysis Unit in the FIMM Technology Centre where he still serves as a senior advisor. In this role, he helps other researchers with image analysis, and plans collaborative projects. Most recently, Dr. Paavolainen is developing a new project that could lead to a wider collaboration with FIMM Group Leader Esa Pitkänen and his team.
Outside of FIMM, Dr. Paavolainen is connected to the European Research Infrastructure Euro Bioimaging, and appreciates the resulting discussions with EMBL, the biological hub of European bioimaging. He also has close links to researchers in Sweden, namely Arne Östman at Karolinska Institutet and Olli Kallioniemi’s group at SciLifeLab.
Connections in the United States feed Dr. Paavolainen’s research program as well. An extended research visit has fostered a collaboration with the imaging platform at Broad Institute (USA) and seeded a fruitful collaboration with Juan Caicedo there. In addition, Dr. Paavolainen has a long term collaboration with Holland Cheng’s group at the University of California at Davis utilizing electron microscopy data on proteins of a virus-like particle for drug and vaccine delivery. One of Dr. Paavolainen’s doctoral candidates is currently working in Davis on this project.
Dr. Paavolainen is first to point out that new ideas are emerging continually thanks to these and other connections on both sides of the Atlantic.
An academic career: from computer science to biology
Scientific training and research in the nearly 15 years since his MSc degree have resulted in an independent principle investigator position for Dr. Paavolainen, and he is appreciative of the opportunities at each step along the way, and humble about his accomplishments.
Coming from a computer science background, he found biology in a serendipitous way with good timing.
I was focusing on software engineering with my Masters degree. I found that image analysis and machine learning were very interesting topics, at a very early stage. It was the opposite of the development of mobile games which was a common field to go into. It just so happened that my Master's thesis supervisor knew a cell biology research group, who were actually looking for, at that time, someone to develop software for visualization and analysis of microscopy data.
With his training in software engineering, Dr. Paavolainen could have followed the path to a software company, but, as he explains:
Instead of going where the big bucks are, I took the risk and went for the science to first develop software and then to start my PhD studies on the research of these methods. And, that led me to a postdoc and more towards health applications. I've been very happy with this decision.
As a PhD student, Dr. Paavolainen’s research on bioimage informatics methods for light and electron microscopy image data led to the development of the open-source BioImageXD software, published in Nature Methods. Being ahead of the curve as a PhD student motivated him:
For three years, I was the only developer of the software. We were doing something that basically no one at that time was doing for 3D image analysis, not just the software development, but also the platform for analysis methods and open collaboration as well.
As a postdoc, Dr. Paavolainen and colleagues entered a Kaggle Community Competition. Kaggle is a large global online data science community providing a platform of machine learning competitions. Almost 3,000 teams globally participated in a competition to automate segmentation of cell nuclei. Dr. Paavolainen describes the experience:
At that time, we were novices in deep learning and its application to image analysis. During the project, we were basically trying everything, exploring different approaches, and communicating with others and learning. And we ended up with a very nice method and in the top 1%.
The impact of the experience was not short-lived, he explains, “it was not only about learning and team effort during that time. It also shaped my future work - how I’m thinking about research and that maybe we could have universal models for many, many tasks that are in the image analysis field.”
An eye toward the future
As a new principal investigator, Dr. Paavolainen is looking forward to a bright future with the five-year Academy of Finland Research Fellowship as a foundation. He describes his vision:
In the next five years, I’ll probably maintain both approaches - technology development and applied research - which is also the reason why I am working at FIMM, as compared to a computer science faculty. I definitely want to keep my focus on cell and tissue microscopy image data analysis and how to best use that data for the selected health applications in the future.
For a variety of reasons, only a small percentage of PhD researchers become independent research group leaders. Dr. Paavolainen elucidates what has allowed him to get to this point in his career:
I'd say the general openness for collaboration. Not only focusing on one specific question that I'm interested in but that maybe no one else is that interested in. The wide collaboration at many different levels, not only within our institute, but also abroad, with many research groups. And, that I'm able to have possibilities for application to many practical questions.
He humbly explains further, “I still learn new things on a weekly basis. Not everything can be prepared for.”
His tips to others who are aiming to transition into a group leader position are fundamental, “I wouldn't be afraid of taking the next step. When you have the chance, and are able to take on the group leader position, then just go for it, and things will happen. So go boldly towards your goal.”
But, as Dr. Paavolainen explains, timing matters a lot as well, “I think that my success so far is partly because my science was very well timed. I knew that these were just very interesting topics in research, in general, and they were becoming more and more relevant. So good timing can be key.”
Making a difference for society
At first glance, Dr. Paavolainen’s work may seem distant from everyday life, but he anticipates that the work will contribute to improved diagnosis and patient outcomes.
And, with such developments, a person can become more engaged in their own health. A person could interact directly with the technology: “consider skin cancer, for instance, and imagine that you could actually use your own mobile phone to take an image and have it analyzed automatically, to say accurately whether there is something that you should be concerned about or not.”
As a final thought, Dr. Paavolainen reminds us of passion in academic research, “when you dig deep enough in any field, you realize how much there is to still be discovered, to be uncovered about basic human biology. This is very motivating, very interesting.”
Brief career summary
Dr. Lassi Paavolainen was initially trained in computer science in the Faculty of Information Technology at the University of Jyväskylä (M.Sc. 2007). He then completed his PhD, also in Jyväskylä, in Professor Varpu Marjomäki’s research group in 2013. After finalizing projects as a postdoctoral researcher in Jyväskylä, he moved to a postdoctoral position at the University of Helsinki in 2015 and in 2017 obtained an Academy of Finland Postdoctoral Researcher grant to continue his work in deep learning for phenotypic profiling. During 2017 he also served as Head of FIMM High-Content Imaging and Analysis unit, responsible for establishment and daily operations of the unit. In September 2021, he began his career as a group leader at FIMM, heading the Bioimage Profiling Group, with support from an Academy of Finland Fellowship.
CONTACT
Dr. Lassi Paavolainen
Academy Research Fellow
lassi.paavolainen@helsinki.fi