Johan Lundin: How AI can help in a healthcare setting
Professor Johan Lundin is a Research Director at FIMM (Institute for Molecular Medicine Finland), University of Helsinki. He is also a Professor of Medical Technology at the Karolinska Institutet. His research specializes in developing and studying technologies to aid diagnostics at the point of care, in particular in research-limited countries. Here, he describes his research and explains how AI (artificial intelligence) can be applied in a medical setting.
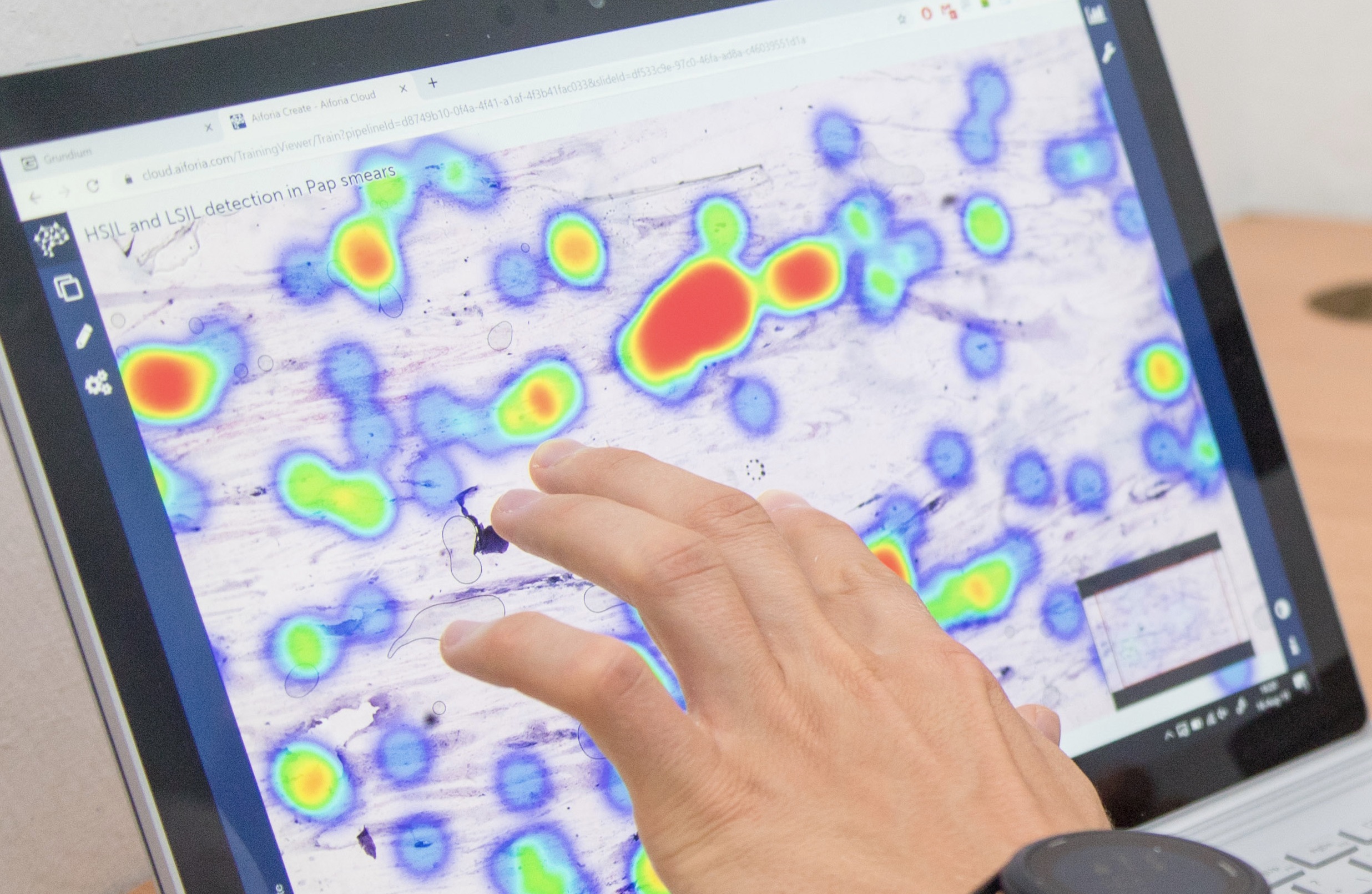
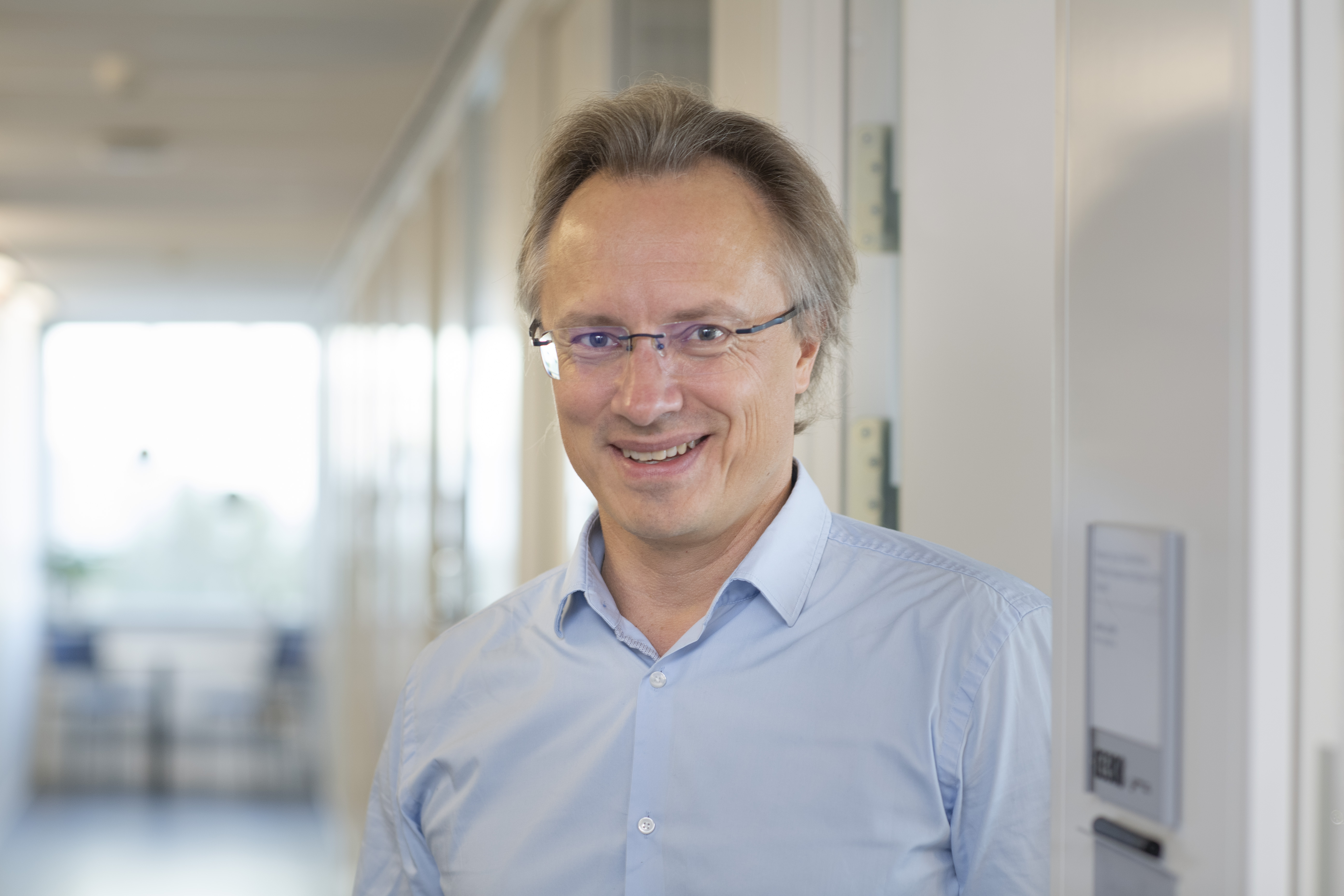
What are your main research aims?
My research examines the use of digital technologies and artificial intelligence (AI) for the improvement of patient diagnostics and care. I aim to develop and study diagnostics that are more accurate and efficient, and that are also safe for the patient. I’m also interested in improving access to diagnostics on a global level.
Have you always worked in this field?
Yes - I actually published articles on AI back in the 1990s; well before it was known as ‘deep learning’ like it is today. The technology was broadly the same but was then referred to as ‘artificial neural networks’. This all came before the so-called ‘AI Winter’ which lasted from the late 1990s until about 2012, when interest and funding in the technology were re-ignited. I have always been interested in the field but regard myself as being a ‘combinator’. When I see something interesting that has been developed, especially where AI or mobile technology is concerned, I try to determine how it could be used for healthcare purposes and research.
In terms of disease areas, my PhD was in cancer biomarkers and my group has also worked on infectious disease diagnostics. The technologies we study are very versatile and have many applications. For example, we have recently looked at classifying burn injuries using AI.
What would you ultimately like to discover?
Ultimately, we would like to be able to make AI-based discoveries. With its recent developments, AI can be described as an extremely powerful pattern-recognizer. It can find patterns that either are not visible to the human eye or patterns that human experts have not yet identified. For example, we recently found that by applying AI to basic morphology, without any antibodies or biomarkers, we were able to predict patients who would best respond (and who would not respond) to a molecularly targeted treatment in breast cancer. These types of patterns might also be spotted by a very experienced pathologist but we were able to prove that we could do the same using AI.
We also want to be able to explain what the AI is seeing in the tissue. Current developments are not so well suited to this. AI is incredibly efficient in learning patterns but it isn’t able to tell us what patterns are relevant, and why they are relevant. We do expect this to improve over time.
Do you think AI will become the default diagnostics tool?
Yes. In the long run, there will be a definite shift in this direction. We already see that AI can go far beyond what a human is capable of in terms of accurate methods of quantifying, discovering, or classifying. However, AI is only useful for very specific tasks. It’s not yet able to take context into account like a human can. It can’t factor in patient history, the local circumstances or the priorities within a certain society’s healthcare system. In that way, AI is quite ‘stupid’; it is limited to ingesting a large amount of data and to quickly finding patterns rather than making ‘clever’ decisions. However, I do believe that its uptake will eventually be very fast, especially in resource-limited countries that lack the medical and technical experts who would otherwise perform these pattern-recognition tasks.
How do you feel people perceive the idea of using AI for diagnostics?
We recently published a study on using AI to help screen for cervical cancer in Kenya. The African countries have very few specialists that can perform cell-based screening; on average less than one pathologist per one million people. In this type of scenario, they might go directly to AI-based diagnostics because it’s so much faster and it makes diagnostics data much more accessible. Interestingly, the acceptance of these methods in resource-limited countries like Kenya is far higher than it is in places like the Nordics. Kenya was one of the first countries in the world to truly embrace mobile banking with their M-Pesa initiative and they appear to be much more accepting of the possibilities offered by technology. High-resource countries have a greater number of healthcare experts available who come with their respected reputations and established domains. I imagine that they will take a little longer to fully embrace AI and similar technologies when it comes to healthcare.
Lab Manager Martin Mulnde scanning PAP smears at the Kinondo Clinic in Kenya. Photo: Oscar Holmström
AI is powerful for seeing things that a human might miss, but this could also cause some issues. For example, if AI can prove that a healthcare expert missed something important, this could open up legal and ethical questions. These will need to be worked through and overcome before the use of AI becomes commonplace. I’m positive about the future though. With the cervical cancer screening project that we ran in Kenya, we are proposing that the AI carries out a screen that a cytotechnologist would usually do. It only screens samples for abnormalities. We can then present the AI findings to a medical expert who will then make the diagnosis, meaning human judgement is still very much involved.
How do you use AI to detect cancer or pre-cancerous cells?
The main method is based on machine learning. Previously, if you wanted to find abnormal cells in an image of a sample, you had to hand-craft the features that were characteristic of an abnormal cell. For example, if the identifier was a big nucleus in the cell or an unusual ratio between the cytoplasm and nucleus, then you had to design an algorithm that specifically measured those attributes. You then needed to compare cells and make a decision.
With machine learning, it’s completely different. You don’t need to know these features, you just need a lot of examples and an expert who knows what they are looking for. An expert marks the abnormal cells in the image, effectively ‘drawing’ to label the abnormalities alongside the normal cells. This then determines the training regions for the AI. The AI uses these labels to try to optimize the classification and find the features that the specialist has marked, thereby distinguishing the abnormal from the normal. It’s also crucial to have a lot of samples that cover the spectrum of all possible variations. This ensures that when new samples are introduced, the AI can continue to detect healthy and abnormal cells. The process involves a lot of active learning and repetition to make sure that there are no errors caused by the original specialist or by the AI encountering something it has not yet learned. This type of iterative process will be needed when doctors use AI for clinical purposes. The technology will require constant revision and improvement but it’s very exciting to think about all the possibilities it will offer.
Could AI be used to make diagnoses in other disease areas aside from cancer?
The technology can be applied to lots of different areas. One important thing to note is that for clinical purposes there are not many AI algorithms currently in practice in the clinic. Some cancer diagnostic analyses algorithms are FDA-approved but they are not so many based on machine learning. We expect this to come in the next two to three years. In other medical fields, AI is already being used, for example for examining the fundus of the eye to detect signs of complications caused by diabetes.
How do you think AI will evolve in terms of its clinical applications, and what challenges do you foresee?
It’s important to note that around 95% of AI is based on so-called ‘expert supervision’. This means an expert is determining what is abnormal and making annotations. The AI then replicates that expert’s performance. That means that if you compared AI-generated classifications made by different pathologists, you will probably get a lot of different results and opinions. The AI is a virtual version of the original expert that trained it.
I see that this could create a big problem. The original purpose of cancer diagnostics and cancer grading is to know more about the outcome of the patient and to guide and plan the treatment. Generating these different opinions in the form of virtual AI-based experts won’t help here. We have, therefore, proposed ‘outcome supervised learning’. Instead of trying to replicate the experts, we want to try to predict outcomes directly. This would mean trying to predict the efficacy of treatment offered, the outcome of cancer, and of course the very endpoint as to whether the patient survives the cancer. We should know more about what treatment was best for the patient in the end, and then use this information to work backwards and predict those outcomes. The outcomes are also more likely to give you opportunities to discover new things. If you keep replicating what is already known then you will never progress or make use of the capacity of machine learning. This is one vision of how to use this more effectively. Knowing the long-term outcomes will take a lot of time and will require large-scale collaborations. I have seen a few groups working on this and I’m sure there will be more.
What do you enjoy most about your research?
I’m very motivated about the possibilities to use new technologies and apply them to the medical domain. It’s very exciting how fast technology develops, it’s just a matter of keeping up and quickly trying to work out how we can apply technological progress to our research.
For example, 5G mobile technology opens up many possibilities. It could mean that an AI expert can work there at the point of care out in the field. Interestingly, these new technologies quickly become accessible in resource-limited countries. During our projects in Kenya and Tanzania, the bottlenecks in our work were caused by time needed for sample preparation rather than connectivity. Sampling can also be hugely improved via affordable technology, for example, we need quality samples in a digital format for them to be useful. Camera improvements are happening all the time and maybe we could use 100 cameras instead of one when it comes to capturing samples digitally. This is simple and affordable and could speed up our work hugely; I am interested in testing out these kinds of scenarios.
As the COVID-19 pandemic is shown, we can change things very quickly when we have to. Once a certain threshold has been passed and people see how beneficial digital technology is for our lives, we very quickly embrace it. The move to hold meetings online over the past year, for example, has been rather effortless. We’ve realized how important technology is and, I believe, we will be quick to do the same where AI is concerned. If something is more accurate, more reliable, and results in better patient outcomes then I think it it is only natural that we embrace it.