Introducing Group Leader Esa Pitkänen
Esa Pitkänen is a FIMM-EMBL Group Leader at the Institute for Molecular Medicine Finland at the University of Helsinki. He specializes in computational cancer genetics, machine learning and bioinformatics. In this interview Esa shares his keen interests and sheds a light on how machine learning and computational analyses can be applied to understand biological questions.
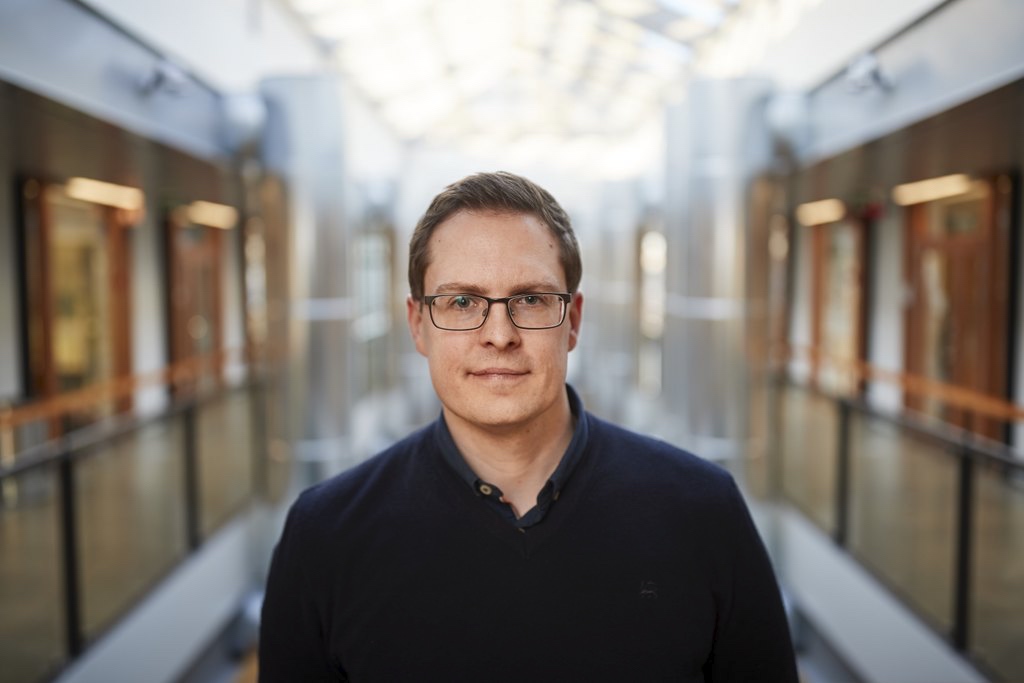
Can you tell me a bit about your background?
Esa comments; “I am a computer scientist by background, and received my PhD from the University of Helsinki in 2010. In my dissertation, I proposed new algorithms for metabolic modelling: some of these methods were co-developed and put into use at VTT Technical Research Centre of Finland for example in creating novel production organisms and to reconstruct metabolic networks for microbes utilized in industrial biotechnology. During this time in addition to research I did also some teaching as the coordinator for the Master’s Degree Programme in Bioinformatics (2006). After PhD, I switched my focus from metabolism to cancer genetics and did a postdoc at the Tumour Genetics research group at the Faculty of Medicine, University of Helsinki. In July 2019, I returned to Helsinki after a three year stay at EMBL Heidelberg, where I was working in the Pan-Cancer Analysis of Whole Genomes project, investigating what role does the germline play in somatic mutagenesis in cancers.”
Can you tell me about your field of interest and research?
Esa is interested in how machine learning and computational analyses can help to find answers to central questions of biology, and transform clinical practice and healthcare. To name a few examples, deep learning approaches in particular have been successful in achieving state-of-the-art results in problems such as cancer classification, genomic accessibility prediction and in silico protein folding. Deep neural networks are machine learning models that became popular a few years ago. These models are powerful feature extractors operating at the level of raw data, but can also integrate and leverage multiple layers of heterogeneous data.
Esa elaborates; “I am interested in taking these techniques and use them to integrate multiple different types of data such as images, genome data and drug treatment response data as another phenotypic layer of data. I thus see deep learning as a key component in utilizing and interpreting various data modalities available in a typical biomedical scenario such as disease diagnostics”.
Deep neural networks are often said to be “black boxes” with the notion that the interpretation of their outputs can be challenging. Fortunately, interpretation of these models is an active research area today, and there is already considerable progress in making sense of the trained models. In biomedical applications this is crucial since any computational prediction must be clearly justifiable for a model to have any chance of being adopted to clinical use. For example, a recent paper shows how deep neural networks is trained to identify skin cancer or skin lesions from photographic images. One can interpret such models for example by extracting the image features which are characteristic to each of the cancer subtypes identified.
Esa has a keen interest in cancer genetics: how do cancers arise? What are the inherited and acquired genetic factors contributing to tumorigenesis? What is their interplay with epigenetic and environmental factors? He comments; “We already know a lot about how cancers arise. A key component are the somatic mutations that cause cancer, giving the cells a growth advantage over the neighbouring cells, giving the cells the ability to invade neighbouring tissue and the ability to metastasize to different parts of the body. However, to fully answer these questions, we need large amounts of high quality, high-throughput data (including environmental factors, epigenetics and genomics) as well as computational methods capable of benefiting from all this data; deep learning and other machine learning techniques are important tools in moving forward towards solutions: instead of writing algorithms we will learn the necessary algorithms directly from combinatory data.”
While there is huge potential applying machine learning to biomedicine, we must also remember the limitations of machine learning. Models can be only as good as the training data - biases present in the data will be present in the models as well.
What question or challenge are you setting out to address with your research?
Integration of multimodal, massive-scale data in biomedicine with machine learning will definitely be one of the overarching themes. Esa will be working under the hypothesis that there is synergy in having more than one view on the object of study, whether that is a novel biological phenomenon or a disease to be diagnosed, for example. To give a bit more specific examples, his team will investigate mechanisms of somatic mutagenesis in various cancer types, phenotypic variation in haematopoietic cells, and genotype-phenotype causality in haematological malignancies.
What are you working on at the moment?
Esa comments; “I am continuing to work on some of the themes I already started at EMBL. One of these is somatic mutagenesis in cancer as already mentioned. The aim is to understand the mutational mechanisms which give rise to somatic mutations which cause and drive cancers, and also play a role in other diseases as well. I believe we can derive insight by jointly analysing multiomics data in cancer, and deep learning will be a powerful tool for achieving this goal.
What translational impact does your research have?
Together with the Helsinki University Hospital Esa (and colleagues) will be actively contributing to the Academy of Finland Flagship project iCAN, which is a platform for cancer research aiming at the development of personalized cancer treatments. The aim is to create a reservoir of all the data that comes from the patients including the computational analyses of this data. This platform will allow them to quickly deploy and evaluate their machine learning methods and models in clinical practice. Esa comments; “We are specifically looking to contribute tools for diagnosis, prognosis and designing treatments in cancer and haematology settings.”
Could you tell me a bit about your group at FIMM?
Our team will initially consist of people with experience in machine learning, computational data analysis and bioinformatics looking forward to solve some of the mysteries of biology and medicine - life in general. “At the moment, our team has four PhD students and a bioinformatician. I’m also looking for a data engineer to join our iCAN project.”
Is it challenging to recruit people in this particular area of science?
Esa comments; “In the field of machine learning, it is difficult to recruit people due to a pull from the industry, there are a lot of opportunities in the industry in data science that are highly attractive, which can be a challenge in academia because we need to provide people with so exciting problems so that they are drawn to solving problems in science. However, I had an open PhD call and received applications from a lot of outstanding candidates from around the world.”
Are you collaborating with other researchers outside FIMM, or within FIMM at the moment?
Esa and his team are collaborating with Dr. Jan Korbel (EMBL Heidelberg) and Dr. Oliver Stegle (DKFZ, EMBL Heidelberg) to develop integrative deep learning techniques for large-scale cancer genetics data especially in the context of the Pan-Cancer Analysis of Whole Genomes project (PCAWG), that includes over 2700 cancers and 39 types of tumours. The PCAWG papers are in press and will published in early 2020. Together with Prof. Lauri Aaltonen and Dr. Pia Vahteristo, within the Applied Tumour Genomics research program of the Research Programs Unit, Faculty of Medicine, they will investigate the utility of these techniques in colon cancers and uterine leiomyomas. At the Meilahti campus, they are working with the Haematological Genetics group led by Dr. Outi Kilpivaara and Dr. Ulla Wartiovaara-Kautto to understand how inherited and acquired mutations contribute to haematopoiesis and haematological diseases. Collaboration with Prof. Kimmo Porkka (Helsinki University Hospital) will aim at developing integrative machine learning approaches for acute leukaemia, aiming specifically at clinical translation of the results.
What are your goals for the first phase as a FIMM-EMBL group leader?
Get the group going and try to create a friendly and accepting atmosphere where people feel free to express their ideas, and enjoy their work. I want to be able to provide my team with the opportunities, resources and support they need to do good science.
Can you share a defining moment in your work as a scientist?
Esa comments; “All the “change points” in my career have had a tremendous impact on how I think about science. I can strongly recommend taking the chance to learn something completely different in a new environment every now and then.”
- Click here to learn more about Esa Pitkänen and his research group.
- Click here to learn more about FIMM.