AI supported diagnostics can facilitate screening for cervical cancer in resource-poor settings
A new study from FIMM group leader, Johan Lundin, at the University of Helsinki and Karolinska Institutet shows that artificial intelligence-supported digital microscopy at the point-of-care can be used to identify atypical Pap smears in a resource-limited setting.
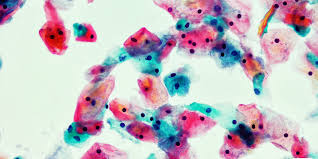
Note: This article originally appeared on the FIMM website.
The team developed and implemented a novel digital diagnostic system at a rural clinic in Kenya, a country where cervical cancer is the leading cause of female cancer-related deaths.
Cervical cancer is highly preventable but remains a common and deadly cancer in countries without screening programmes. Inadequate access to microscopy and molecular diagnostics is a major problem in low-resource areas and impairs the diagnosis of this as well as many other conditions.
An international research team led by Professor Johan Lundin has focused on studying devices and applications that enable artificial intelligence (AI) supported automated point-of-care diagnostics. The group has previously demonstrated the potential of such approach in detecting different parasitic infections.
Novel automated diagnostic tools are greatly needed
In their new proof-of-concept study, the group aimed to study whether AI supported diagnostics performed via a remote connection could facilitate screening for cervical cancer in Sub-Saharan Africa.
According to Johan Lundin, there is a great need for novel automated diagnostic tools. The project uses AI for diagnostics in an area where there are limited resources and in average less than one pathologist for a million people.
“Conventional Pap smear analysis can drastically reduce the incidence and mortality of cervical cancer, but the manual analysis of samples is labour intensive, prone to variations in sensitivity and reproducibility, and requires medical experts to analyse the samples, thus making it hard to implement in resource-limited settings”, he said.
Field studies essential for testing the method in rural, resource-limited settings
In cooperation with the Kinondo Clinic located in a rural area south of Mombasa in Kenya, the team collected Pap smears from 740 women and digitized them with a portable scanner. After scanning, the whole-slide images were uploaded to a cloud server using mobile networks and analysed with a deep learning system.
The researchers used about half of the collected samples to train the deep learning algorithm and the rest to test its performance for the detection of atypical cervical cells. The results were then validated by comparing them with the visual assessment of the physical samples by a local pathologist and evaluation of the digital samples by a cytotechnologist and a pathologist in Finland.
The results remonstrated that AI supported automated diagnostics performed well in detecting abnormal samples, especially in the case of moderate and severe precancerous lesions. In these cases, the deep learning system correctly detected 96-100% of abnormal samples, as compared to the visual evaluation of the samples by the pathologist. The abnormal findings can then be confirmed by a pathologist and the lesions treated for example by cryotherapy in order to prevent a cervical cancer from occurring.
“To our knowledge, our study is the first one where digital whole slide images of entire Pap smears were captured and analysed with AI at the point-of-care in a rural, resource-limited environment”, says the first author of the study, Oscar Holmström from the Institute for Molecular Medicine Finland FIMM at the University of Helsinki.
Since the results of the study demonstrated that the method developed in this work can reliably classify those samples that are normal, clinical implementation of the method could significantly reduce the sample analysis workload and thus allow pathologists to focus on verifying the potentially abnormal results. In Kenya only five per cent of women currently have access to cell-based screening for cervical cancer, and vaccination against the human papillomavirus (HPV) to prevent cervical cancer is still not widely available.
“The fact that we achieved high accuracy for the detection of atypical slides in these demanding settings, suggests that the method may be useful for screening purposes in resource-constrained settings”, says Associate Professor Nina Linder from the University of Helsinki, who co-supervised the study.
Original publication: Point-of-care digital cytology with artificial intelligence for cervical cancer screening in a resource-limited setting. Oscar Holmström, Nina Linder, Harrison Kaingu, Ngali Mbuuko, Jumaa Mbete, Felix Kinyua, Sara Törnquist, Martin Muinde, Leena Krogerus, Mikael Lundin, Vinod Diwan, Johan Lundin. JAMA Network Open, doi: 10.1001/jamanetworkopen.2021.1740
Fundind and disclosures:
Two of the study’s authors, Johan Lundin and Mikael Lundin, are the founders and co-owners of Aiforia Technologies Oy, whose machine learning and image analysis platform was used in the study for developing the deep learning system.
The research was supported by grants from the Erling-Persson Family Foundation, the Swedish Research Council, the Sigrid Jusélius Foundation, the Finnish Society of Medicine, Medicinska Understödsföreningen Liv och Hälsa, the K. Albin Johansson Foundation, the Perklén Foundation, the Wilhelm and Elsa Stockmann Foundation and the Biomedicum Foundation. The Finnish company Grundium contributed by donating one of the scanning instruments used in the study.
More information
Johan Lundin, Research Director, Institute for Molecular Medicine Finland (FIMM), HiLIFE, University of Helsinki
and Professor of Medical Technology, Department of Global Public Health, Karolinska Institutet, Stockholm, Sweden
Tel: +358 50 415 5459, e-mail: johan.lundin@helsinki.fi
</article>